Let’s dive into a topic that’s pretty crucial but often overlooked – understanding different types of data. We’re surrounded by data everywhere, in this digital era, and it’s like the backbone of modern business insights.
Whether you’re crunching numbers for a market analysis or predicting the next big trend in data science, knowing your data types is like having a secret weapon.
Imagine data types as different flavors in a chef’s kitchen. Each type has its unique taste (or in our case, use) and knowing which flavor to use can make or break a dish (or a data project). From the bustling world of digital business to the intricate workings of statistics and market research, data types are the silent heroes.
Data types come in two main categories – qualitative and quantitative.
Think of qualitative data as the descriptive, more subjective type. It’s like the color commentary in a sports match, adding context and color.
Quantitative data, on the other hand, is all about numbers and measurements – the scoreline of the match, if you will. Both types are critical in decision-making and data analysis.
They’re like the yin and yang of the data world, each playing a unique role in painting the full picture.
Qualitative vs Quantitative Data
Okay, let’s get into the nitty-gritty of these two main players in the data game.
Definition and Key Differences
Qualitative data is all about descriptions and characteristics. It’s the kind of data that tells a story, often collected through interviews, observations, or surveys. Think of it like capturing the essence of something rather than measuring it.
Quantitative data is the total opposite. It’s all about numbers and stats. This is the data you can easily measure and express in numerical terms – like how many people visited your website or the percentage increase in sales last quarter.
Characteristics of Qualitative Data
Qualitative data is all about the ‘quality’ of information. It’s subjective and often includes:
- Textual data like interviews or open-ended survey responses
- Observations that capture feelings, opinions, or behaviors
- Non-numeric data that gives insights into experiences and motivations
It’s like being a detective, looking for clues in words and behaviors to solve a puzzle.
Characteristics of Quantitative Data
Quantitative data, on the other hand, is the ‘quantity’ part of the equation. This type includes:
- Numerical data like sales figures or test scores
- Data that can be measured or quantified objectively
- Statistics that help in making predictions or identifying trends
It’s a bit like being a mathematician, dealing with numbers and formulas to find answers.
Qualitative Data Types
Nominal Data
Nominal data? Think labels and names.
It’s like sorting your playlist by genre, not by how many times you’ve played each song.
This data type is all about categorization without any order or rank. You’ve got your apples and oranges, but you’re not saying which is better.
- Characteristics: It’s like tagging – you put things in boxes with labels. Red, blue, green; cat, dog, hamster – you get the idea.
- Examples and Applications: Think of a survey asking your favorite type of music. Rock, Pop, Jazz – that’s nominal data. In market research, this is super handy for segmenting customer preferences or demographic info.
- Additionally, customer data platforms are instrumental in organizing and interpreting nominal data, allowing businesses to build comprehensive profiles that enhance targeted marketing strategies.
Ordinal Data
Now, let’s turn to ordinal data. This one’s about order. Imagine rating your top five movies. It’s still qualitative, but there’s a clear first, second, third, and so on.
- Characteristics: It’s qualitative but with a sense of ‘more’ or ‘less’. However, the exact difference between ranks isn’t clear.
- Examples and Applications: Think of a feedback form with options like ‘satisfied’, ‘neutral’, ‘dissatisfied’. In customer satisfaction analysis, this type of data is a goldmine.
Comparison: Nominal vs Ordinal Data
So, nominal is like sorting books by genre and ordinal is like ranking your top 5 TV shows. Both are about qualities, but ordinal data adds a layer of hierarchy.
- Key Differences and Similarities: Nominal data is all about categorization without inherent order. Ordinal data, however, introduces a rank or order. They both fall under the umbrella of qualitative data types and are crucial in fields like data categorization methods and understanding customer behaviors.
Quantitative Data Types
Now, let’s switch gears to quantitative data types. This is the realm of numbers and measurements – the concrete stuff.
Discrete Data
First up, discrete data. This one’s like counting marbles in a jar. It’s all about things you can count on your fingers (or toes, if you’re out of fingers).
- Characteristics: Think of whole numbers. You can’t have half a car or 2.7 children, right?
- Examples and Applications: In statistical data characteristics, you use discrete data to count things like the number of customers in a store or the number of posts on a blog.
Continuous Data
Next, let’s talk about continuous data. Imagine measuring the length of a ribbon or the time it takes to run a marathon. This data can take any value within a range.
- Characteristics: It’s all about measurements that can be infinitely divided. Think decimals and fractions.
- Examples and Applications: In data science basics, continuous data helps in precise measurements like temperature, speed, or weight.
Comparison: Discrete vs Continuous Data
So, discrete data is like counting apples, and continuous data is like measuring honey pouring out of a jar. One is countable, the other measurable.
- Key Differences and Similarities: Discrete data is countable and often whole numbers. Continuous data is measurable and can be any value within a range. Both are integral in quantitative data analysis, from business intelligence to scientific research, where numbers tell the story.
Advanced Data Types
Interval Data
Think of interval data as the sophisticated cousin in the data family. It’s all about the difference between values, but there’s no true zero point.
It’s like measuring temperature. Zero degrees doesn’t mean no temperature, right?
- Characteristics: Interval data is numeric. The distances between values are meaningful, like the difference in degrees between 30°C and 40°C.
- Examples and Applications: This shines in statistical data characteristics and market analysis. Like tracking temperature changes over a week or survey responses on a scale from 1 to 5.
Ratio Data
Now, let’s talk ratio data. This one’s the full package. It’s got a true zero and can tell you about the relative size of things. Like, how many hours you actually slept last night.
- Characteristics: It’s quantitative, with a true zero point. This means you can talk about how many times more or less one thing is compared to another.
- Examples and Applications: In areas like data processing and categorization, ratio data helps in precise measurements. Think weight, height, or time spent on a website.
Challenges and Considerations in Data Classification
Overlap and Distinctions Among Data Types
The lines between data types can get blurry. Sometimes, what looks like nominal data could play the role of ordinal data. It’s a bit like mistaking a zebra for a horse because of its stripes.
- Handling Mixed Data Types: Imagine juggling apples and oranges while riding a unicycle. That’s what handling mixed data types feels like in big data types or data mining.
- Importance of Accurate Data Classification: Getting your data types wrong is like using salt instead of sugar in a cake. It can lead to misinterpretation of data, especially in fields like predictive analytics and data interpretation techniques.
Data Types in Statistical Tests
Choosing the right statistical test based on your data type is crucial.
Selection of Appropriate Tests Based on Data Type: It’s about matching the test to the data. Use a chi-squared test for nominal data, or a t-test for ratio or interval data.
It’s all about finding that perfect fit, ensuring your analysis in fields like machine learning data requirements or statistical data characteristics is on point.
Your beautiful data deserves to be online
wpDataTables can make it that way. There’s a good reason why it’s the #1 WordPress plugin for creating responsive tables and charts.
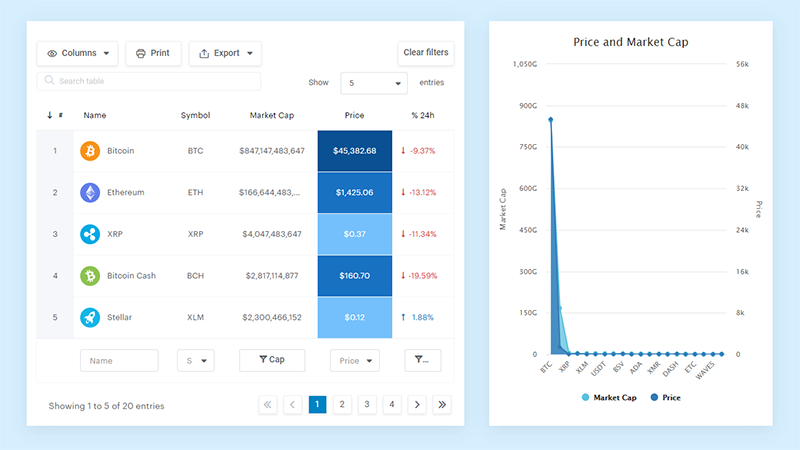
And it’s really easy to do something like this:
- You provide the table data
- Configure and customize it
- Publish it in a post or page
And it’s not just pretty, but also practical. You can make large tables with up to millions of rows, or you can use advanced filters and search, or you can go wild and make it editable.
“Yeah, but I just like Excel too much and there’s nothing like that on websites”. Yeah, there is. You can use conditional formatting like in Excel or Google Sheets.
Did I tell you you can create charts too with your data? And that’s only a small part. There are lots of other features for you.
FAQ on the Different Types of Data
What’s the Difference Between Qualitative and Quantitative Data?
Qualitative data is all about descriptions and perceptions. Think of it like capturing a story. It dives into understanding ‘why’ and ‘how’.
Quantitative data, on the flip side, is about numbers and measurements. It’s more ‘what’ and ‘how much’.
While qualitative data explores attitudes, feelings, and behaviors, quantitative data provides statistical insights, often used in mathematical and statistical analysis. Both are key in comprehensive research, offering different lenses to view the same scenario.
Why are Different Types of Data Important in Research?
Different types of data are like tools in a toolbox. Each has its unique purpose. In research, using a mix of data types allows for a more rounded understanding.
Qualitative data brings depth, understanding the ‘why’ behind behaviors. Quantitative data brings breadth, offering measurable, objective insights.
Together, they enrich research findings, making them more robust and reliable. This combination is vital in fields like market analysis, psychology, and social sciences.
How Can I Tell if Data is Nominal or Ordinal?
Nominal data is about labeling without a specific order. Think of it as naming or categorizing items. For instance, types of cuisine (Italian, Chinese, Mexican) are nominal.
Ordinal data, however, has a clear order or rank. An example would be a satisfaction survey with ratings like ‘Poor’, ‘Fair’, ‘Good’, ‘Very Good’, ‘Excellent’.
There’s a distinct hierarchy, but the exact difference between these ranks isn’t numerically defined.
What Are Examples of Discrete and Continuous Data?
Discrete data is countable, usually whole numbers. Imagine counting the number of cars in a parking lot. It’s discrete because cars are countable units.
Continuous data, conversely, can take any value within a range and can be divided infinitely. Think measuring the length of a ribbon.
It can be 2 meters, 2.05 meters, or even 2.057 meters. Discrete data is about counting; continuous data is about measuring.
In What Ways are Interval and Ratio Data Different?
Interval and ratio data are both types of quantitative data, but there’s a key difference. Interval data has no true zero point.
A great example is temperature. 0°C doesn’t mean there’s no temperature. Ratio data, on the other hand, has a true zero point and allows for comparison of magnitudes.
Weight is a ratio data example. 0 kg means there’s no weight, and you can say something is twice as heavy as another.
What Role Do Data Types Play in Statistical Testing?
Data types determine which statistical tests to use. For example, nominal data often uses chi-square tests, while ordinal data might use non-parametric tests.
Interval and ratio data are suited for parametric tests like t-tests or ANOVAs. Choosing the right test is crucial for accurate results.
It’s like picking the right key for a lock – using the wrong one can lead to misleading conclusions. It’s all about matching the data type with the appropriate statistical method.
How Does Understanding Data Types Improve Data Analysis?
Understanding data types is essential for effective data analysis. It’s like knowing which glasses to wear for reading versus distance.
Each data type provides a different view and requires specific analytical approaches. Qualitative data offers depth and insight into behaviors and perceptions, while quantitative data provides measurable and comparable facts.
Knowing how to analyze these correctly ensures accurate, insightful conclusions, enhancing the quality of research, decision-making, and strategy development.
What Challenges are Faced When Classifying Data?
Classifying data can be tricky, like sorting a mixed bag of nuts. Challenges include determining the correct type for each data set, especially when data exhibits characteristics of multiple types.
Handling mixed data types requires careful consideration to ensure accurate analysis and interpretation. Another challenge is ensuring data quality and integrity.
Misclassification can lead to flawed analysis and decisions. It’s a careful balance of understanding the nature of the data and applying the appropriate classification methods.
Conclusion
From the get-go, diving into the world of data types is like stepping into a maze. You’ve got your qualitative data, painting pictures with words and feelings.
Then there’s quantitative data, where numbers rule and everything’s measurable.
It’s a bit like being in a library where one section is all colorful storybooks (hello, qualitative data), and the other is filled with encyclopedias and almanacs (quantitative data, for sure).
If you liked this article about different types of data, you should check out this article about categorical data examples.
There are also similar articles discussing nominal vs ordinal data, discrete vs continuous data examples, descriptive statistics, and how to present data visually.
And let’s not forget about articles on real-time data visualization, primary data vs secondary data, advantages of secondary data, and data visualization principles.